
AI for iRecovery® System: Leveraging Digital Technologies to Improve Sustainable Steelmaking – Alessandro Croci
YML Contest for the 2021 WM Report
From March to July 2021, the YML Contest for the 2021 World Manufacturing Report was held, inviting young leaders from all over the world to submit a case study relevant to the topic of Digitally Enabled Circular Manufacturing. The submissions were evaluated by the World Manufacturing Foundation and the winning case studies are included in the publication.
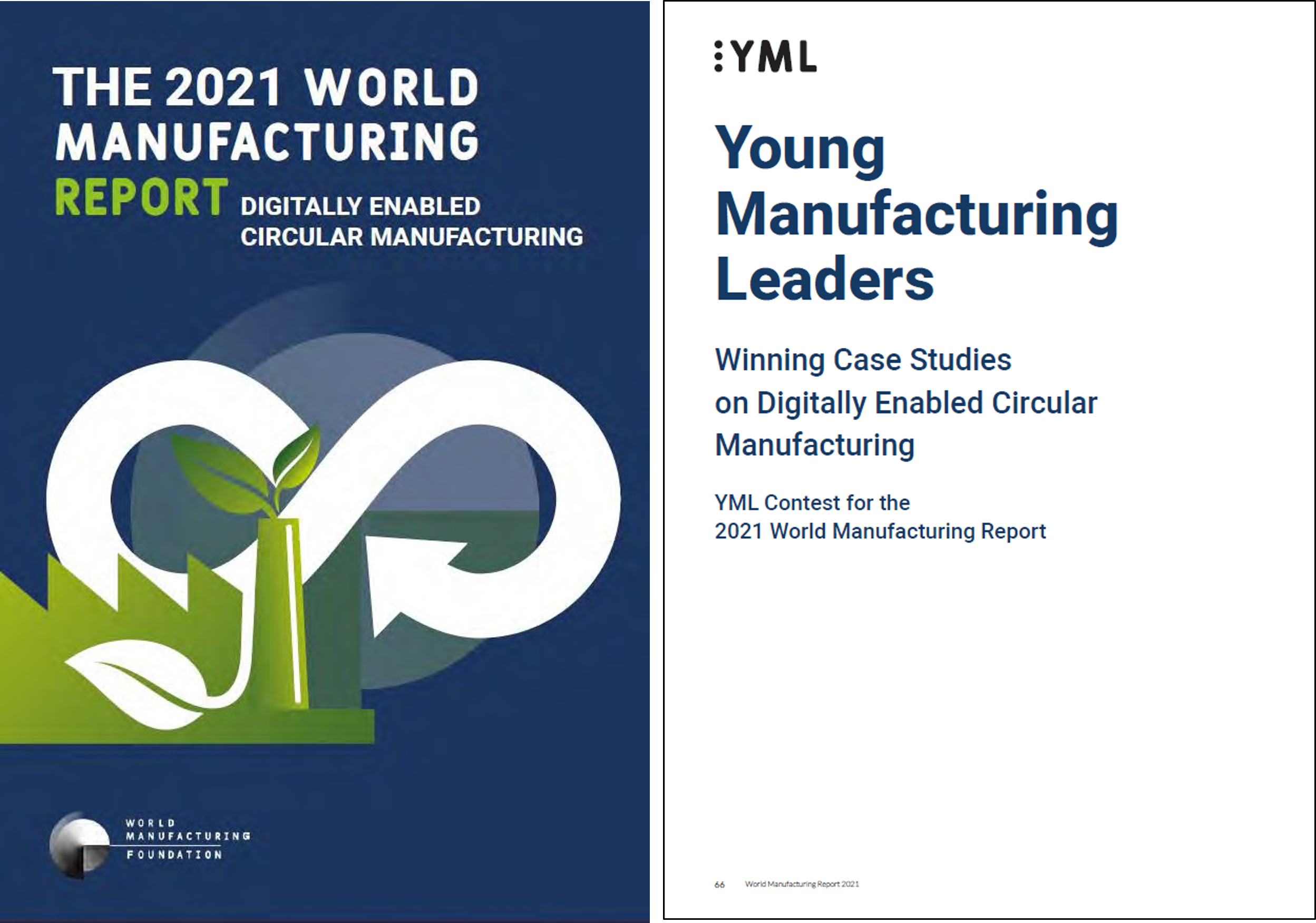
AI for iRecovery® System: Leveraging Digital Technologies to Improve Sustainable Steelmaking
Alessandro Croci, Digital Software Engineer at Tenova, YML Milan City-Hub
Tenova is a worldwide partner for the development of sustainable, innovative and reliable solutions in the metallurgical and mining industries. As such, we provide our customers with a fully integrated range of products, innovative technologies and high-quality services in the field of metalworking, hot stamping, heat treatment and cold rolling. We are active at the forefront of the ecological transition and digitalisation process; the strategic importance of these two processes has led us to coin a neologism, “Sustenovability”, to denote our resolution to provide tech solutions enabling the green transition of the metals industry.
Closely coupled to sustainability is the concept of the circular economy, a disruptive change of paradigm from the linear economy, where the “take, make, dispose” model is replaced by a “reuse, remanufacture, recycle” pattern. An important role in the circular economy is played by the valorisation of waste materials, along with the reduction of the resources needed to manufacture products and the related environmental impact of those processes.
In the steel industry, these concepts are not new: steel can be recycled, without loss of quality, over and over, an infinite number of times. Indeed, steel is the most recycled material in the world, with 650 million tonnes of steel recycled every year.1 This is exactly what happens when steel is produced by an electric arc furnace (EAF) since its main input is recycled scrap metal coming from very different sources. The EAF uses electricity as its primary energy source, drastically reducing emissions of greenhouse gases and pollutants in the environment. Proceeding towards a zero-waste approach, Tenova has developed a technology, named the iRecovery® System, which has the ambitious goal of recovering the thermal energy contained in the fumes generated during the scrap melting and superheating process in the electrical furnace. The energy extracted from the fumes by the iRecovery® System is used to produce steam, which is made available to transfer the recovered heat to any thermal users. In the Italian city of Brescia, the thermal energy recovered by fumes and converted into steam is firstly stored and then transformed, during summertime, into electrical energy through an ORC (Organic Rankine Cycle) turbine, or, during wintertime, into thermal energy, feeding a local district heating network. This is a closed-cycle system that does not waste water and can recover up to 75% of the energy that would normally be lost. The energy recovered in this way is able to provide around 2,000 households with thermal heat during winter, while during summer, 700 families can rely on clean electricity. Overall, the community benefits from a reduction of 10,000 tons of CO2 per year.
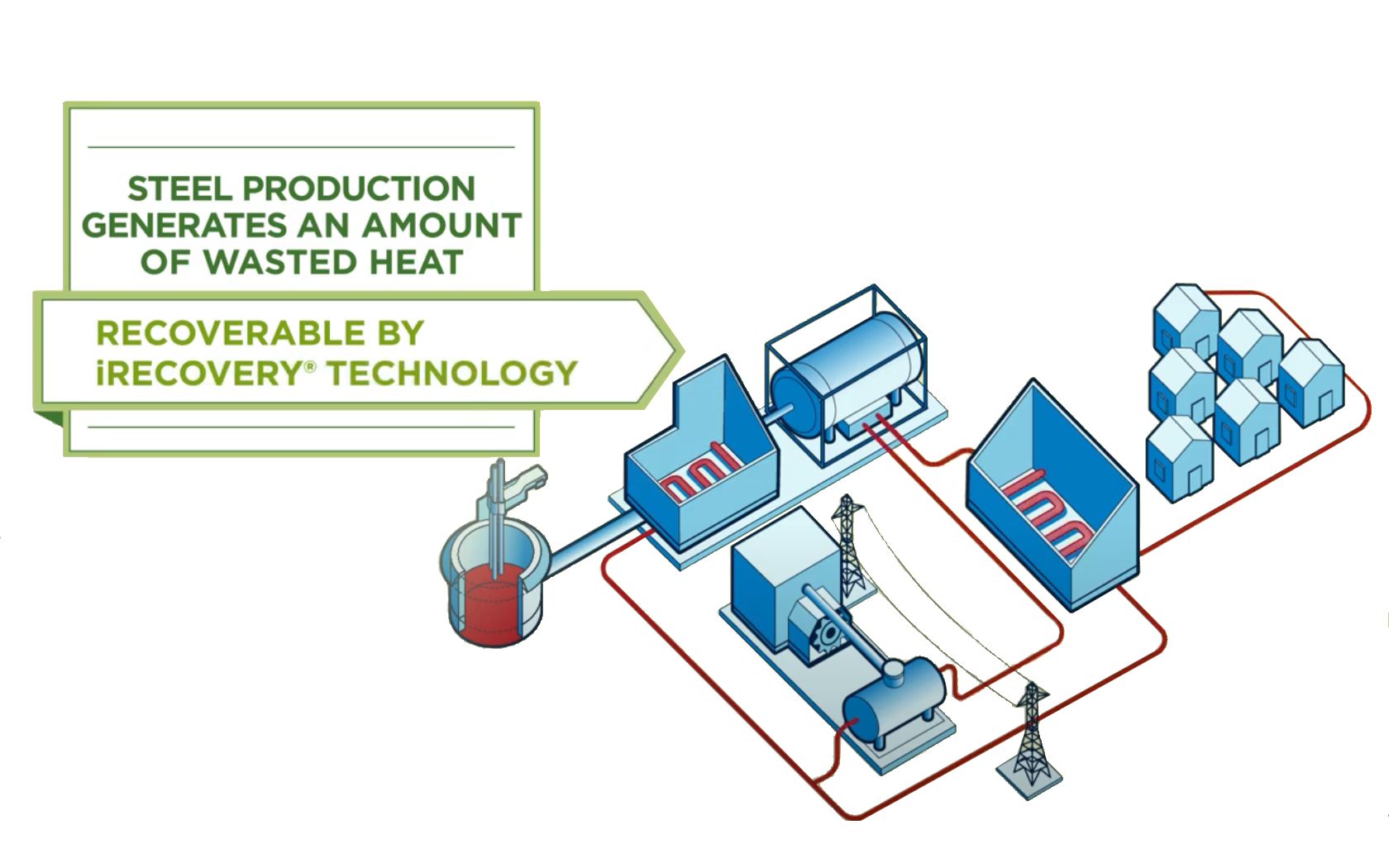
Leveraging the digital transformation taking place within the Industry 4.0 revolution, we are striving to further enhance the performance of the iRecovery® system. Our goal is to predict the mean thermal energy provided by the electric arc furnace over time, as a function of how the melting process is operated, using machine-learning techniques to develop a predictive model.
This model will address the high discontinuity of the melting process, helping to provide a steadier energy flow to the ORC turbine and district heating unit. By forecasting the value of the available thermal energy, we can optimally set up the controller by regulating the amount of power delivered. This will result in an increase in the overall efficiency of the system, which will be able to recover, transform and deliver more energy.
This project will make use of Artificial Intelligence models, learning the correlations between the input features, characterising the melting process, and the target, the steam production, from plant data. Just like any other data science project, the modelling phase is not the most complicated part. A machine-learning model is of no use if it is not properly surrounded by an architecture that is able to respond to different needs, such as supplying data to the model in order to produce the desired predictions, feeding them into the automation control system that regulates the operation of the industrial process, while monitoring the model continuously and retraining it periodically, so as to maintain high and consistent performance over time. Consistency of performance is, in fact, one of our main concerns when designing a new machine-learning project, especially in a sector such as manufacturing: a model should always perform well, in spite of changing operating conditions, modifications to the equipment, or other variable exogenous factors. The only way to address this variability is through accurate model monitoring and retraining. Underestimating the importance of having such an architecture when building machine-learning products is, in my opinion, one of the causes of the extremely high rate of AI projects in the industry that have failed to meet company expectations, hovering around the 90% mark.2 On the other hand, developing the architecture surrounding an ML model is complex and expensive; doing this for every different project is simply neither advantageous nor scalable.
For this reason, it is advisable to create a machine-learning life cycle management system, referred to as “MLOps”, that automatically manages these aspects for every data science service deployed to a customer, similar to the way in which “DevOps” has become a common practice for traditional software management.
In order to foster the development of digital and data science projects, we developed the Tenova IIoT (Industrial Internet of Things) Platform, which addresses all the requirements stated above. This is done with the help of the TenovaEdge, an edge computer installed at our customer’s plant that gathers real-time data from our machines and transmits it to the Tenova cloud platform with the highest security standards, where the data is stored. On our platform, data is transformed in such a way as to be used to train and improve our machine-learning models; when their performance meets our requirements, the model can be deployed to the customer. The model is fed with real-time data and the resulting predictions are exploited to fulfil the model’s scope, such as monitoring an industrial process, performing anomaly detection and predictive maintenance tasks, or tuning the set-points of our automation systems.
In a company like Tenova, where the focus has always been on providing technologies able to take the metals industry in the direction of sustainable manufacturing and carbon neutrality, digitalisation and Industry 4.0 can represent the right set of tools to accelerate this transition and meet governments’ goals, like the European Commission objectives fixed with the Green Deal for 2030 and 2050.3
References
1. Deloitte. (2019). Deloitte Survey on AI Adoption in Manufacturing. Retrieved from: https://www2.deloitte.com/cn/en/pages/consumer-industrialproducts/articles/aimanufacturing-application-survey.html
2. Eurofer. A Green Deal on Steel. Retrieved from: https://www.eurofer.eu/publications/ position-papers/a-green-deal-on-steel-update
3. World Steel Association. Steel Recycling. Retrieved from https://www.worldsteel.org/ steel-by-topic/sustainability/materiality-assessment/recycling.html